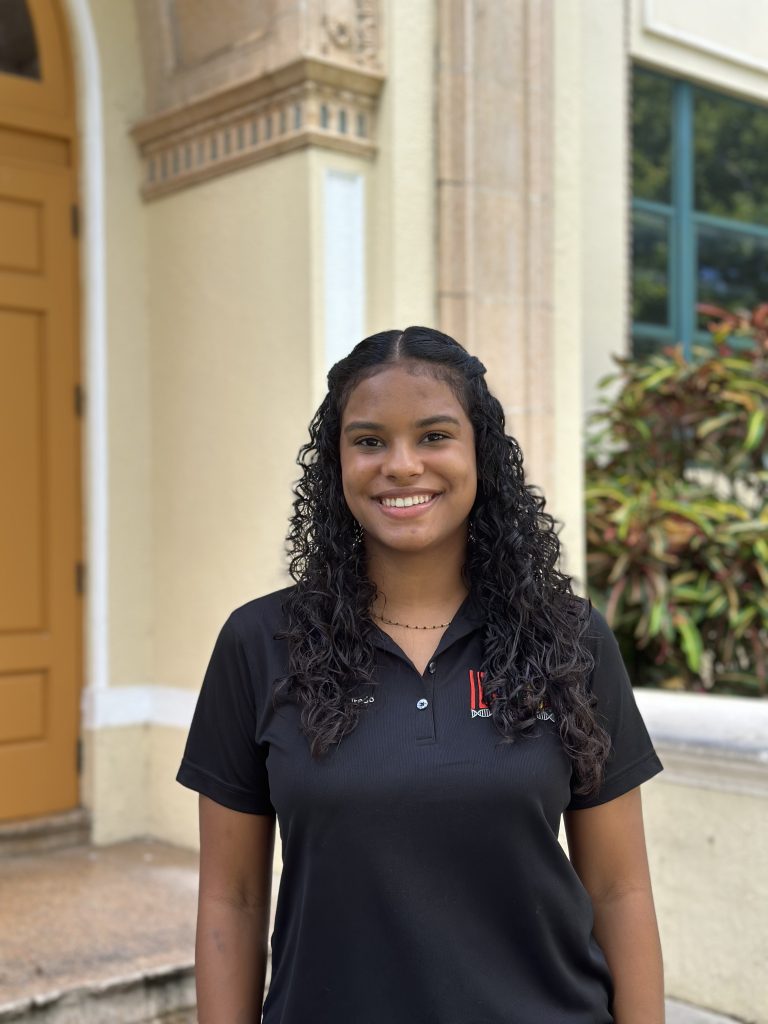
Home Research
Mentor/s:
José E. García Arrarás, PhD
Project Title:
An alternative view of the role of inflammation in intestinal regeneration
Project Title:
Inflammasome is a multiprotein complex that plays a crucial role in the innate immune system by regulating inflammatory responses to cellular stress or damage. Inflammasomes are primarily composed of NLRP receptors, which detect pathogen-associated molecular patterns (PAMPs) or damage-associated molecular patterns (DAMPs). The NLR (NOD-like receptor) superfamily, comprising 22 human genes, is integral to this process. These genes contain a nucleotide-binding domain (NACHT), present in various proteins involved in regulating programmed cell death. The present research aims to investigate the role of inflammation in regenerative processes. To achieve this, we use bioinformatics and experimental protocols applied to a model system of intestinal regeneration, the sea cucumber Holothuria glaberrima. Genes associated with inflammation were identified in transcriptomic database from different regenerative stages. Preliminary findings indicate the presence of NLRP family genes, including NLRC5, NLRC4, NLRP6, NLRP7, and NLRP10 in the intestinal tissue. Surprisingly, the expression of these genes was down regulated during the first two weeks of intestinal regeneration, suggesting that inhibition of inflammation is necessary for the intestinal regeneration process in H. glaberrima. Ongoing experiments use inflammation inhibitors (Diclofenac) and activators (LPS) to modulate the inflammation in regenerative animals. Thus, we provide the foundation for understanding the relationship between inflammation and regeneration in H. glaberrima, with broader implications for regenerative biology and immune system interactions.
Summer Research
Mentor/s:
YeYe
Project Title:
Enhancing Influenza case detection from electronic health records using Large Language Models
Project Title:
Large language models (LLMs), such as Llama, show significant potential in biomedicine. This study leverages advanced natural language processing techniques to enhance infectious disease case detection from clinical data. Specifically, we studied zero-shot identification of influenza cases from emergency department visits using tabular data. In a previous study, we retrieved patients’ ages and clinical findings from electronic health records (EHR) and saved this information into an Excel table. The data were meticulously collected and organized, encompassing numerous patient records annotated for various symptoms and their presence or absence. This table does not contain any patient-identifiable information. Using the tabular data and variable descriptions, we created natural language strings and a short description of the classification problem. With these generated prompts, we queried LLMs, such as Llama, to estimate the probability of having influenza. We explored different prompt generation strategies and compared their accuracy, demonstrating that LLMs can enhance EHR-based case detection capabilities.
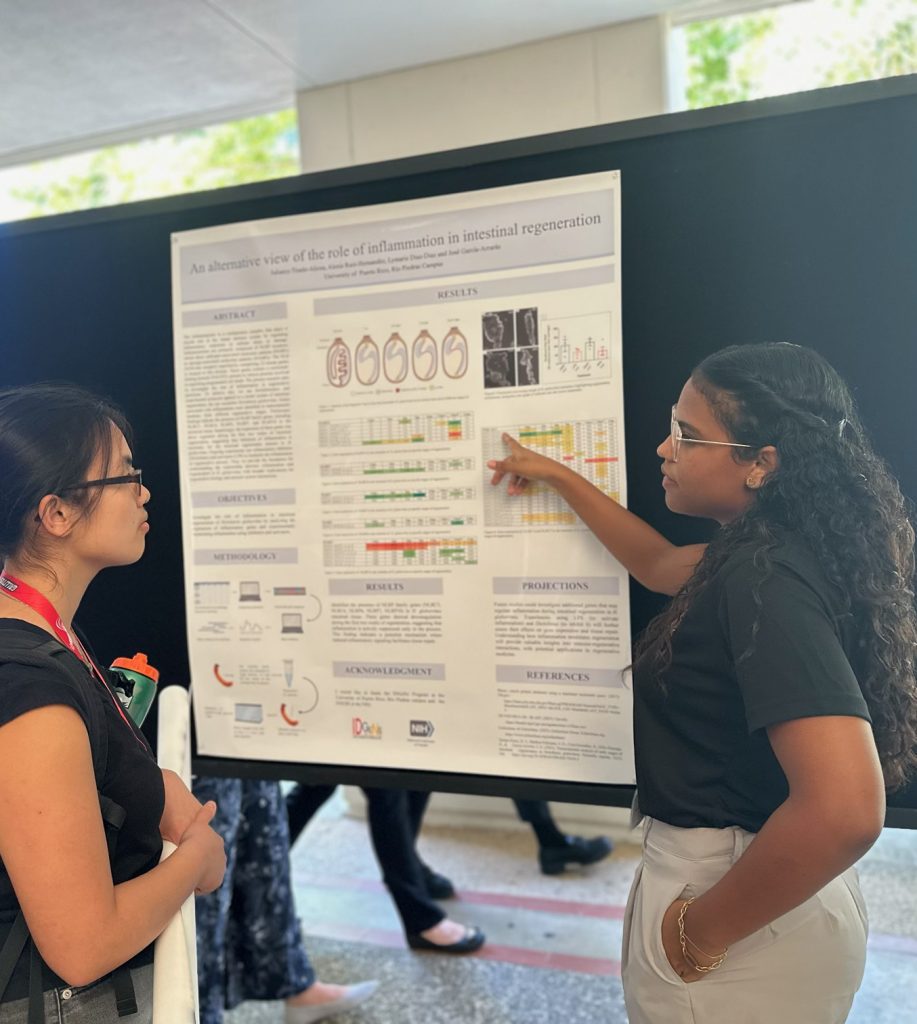